Lessons Learned from Algorithmic Impact Assessments in Practice

TL;DR Understanding algorithmic impact is critical to building a platform that serves hundreds of millions of listeners and creators every day. Our approach includes a combination of centralized and distributed efforts, which drives adoption of best practices across the entire organization — from researchers and data scientists to the engineer pushing the code.
How we approach algorithmic responsibility
At Spotify, our goal is to create outcomes that encourage recommendation diversity and provide new opportunities for creators and users to discover and connect. Thisalsomeans that we are taking responsibility for the impact that we have on creators, listeners, and communities as a platform, and ensures we can assess and be accountablefor their impact. To achieve this goal, we evaluate and mitigate against potential algorithmic inequities and harms, and strive for more transparency about our impact. Our approach is to monitor Spotify as a whole, as well as enable product teams, who know their products best, to optimize for algorithmic responsibility.
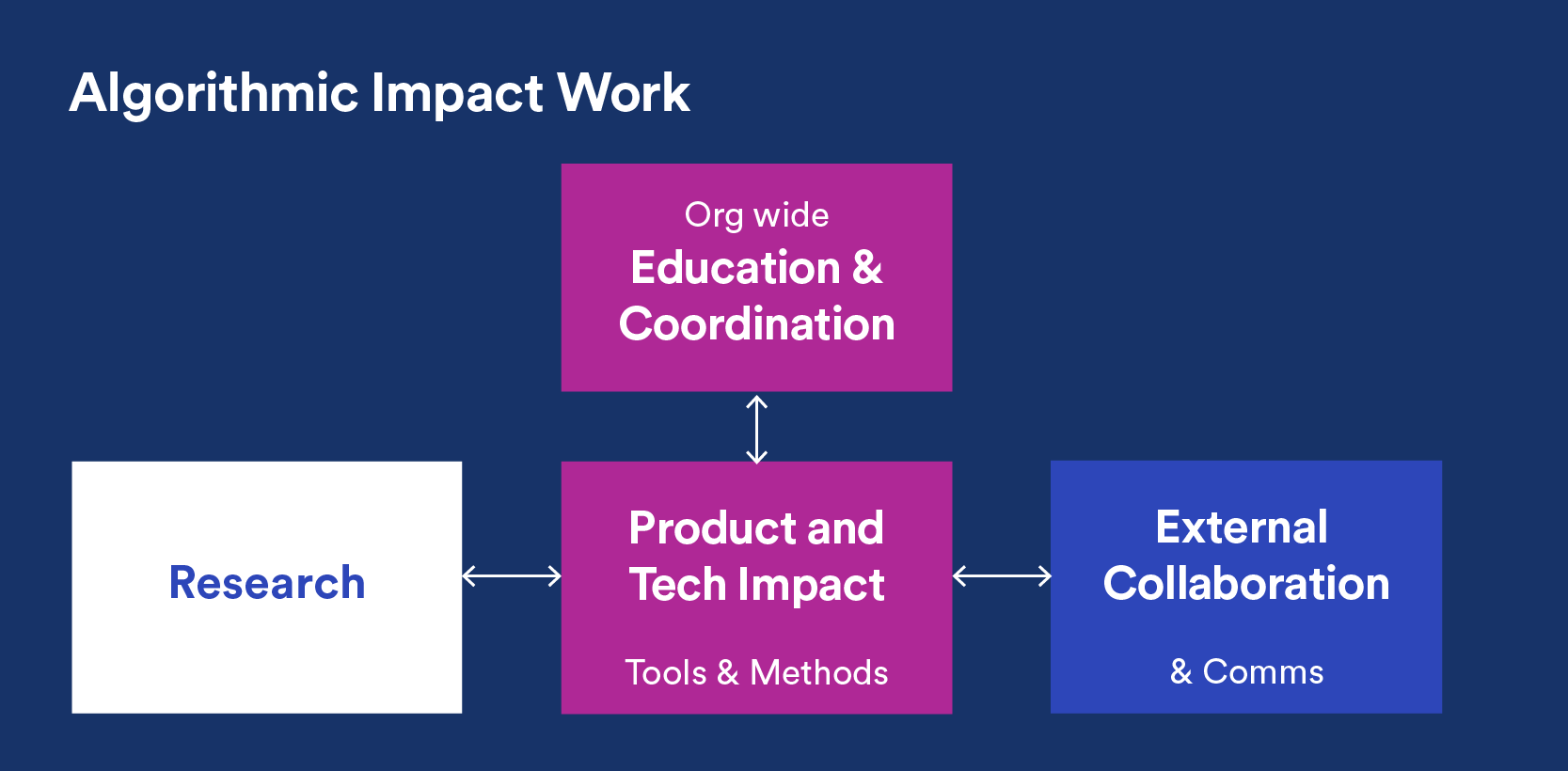
Broadly, Spotify’s approach to algorithmic responsibility covers three areas:
Research: How we combine our research methods across disciplines with case studies to ensure high-quality data decisions and equitable algorithmic outcomes.
Product and tech impact: How we work with our product teams to determine which changes can actually address algorithmic impact in practice. This requires creating awareness of challenges and opportunities to advance responsibility in our products through education and coordination.
External collaboration: How we share and collaborate with other researchers and practitioners in the community at large.
Research
From privacy-aware deep dives into metrics and methods to product-focused case studies, our algorithmic responsibility research, which we’ve been doing for several years, serves as the foundation for understanding the issues that we’re most concerned with at Spotify.
Some examples of our published research include: investigating representation of female artists in streaming (2020), assessing the accessibility of voice interfaces (2018), exploring how underserved podcasts can reach their potential audience (2021), and reflecting on the challenges in assessing algorithmic bias itself (2018).
Product and tech impact
Learning from research has to be translated into tools and methods that work in practice. This requires direct collaboration with product teams.
For example, the algorithmic impact research community has begun offering ML fairness toolkits, which aim to turn the latest fairness research into APIs that anyone can use. When these first appeared, our researchers were excited about the potential of this new class of tool and worked together with our product teams to evaluate which ones could work with Spotify’s recommendation systems. As a result of this collaboration between research and product, we found that a continued effort is required for these research toolkits to be effective from a practical standpoint including where they needed the most improvement.
Advancing algorithmic responsibility requires domain experience and expertise to be useful in the real world. We achieve this through organization-wide education and coordination. Researchers in our algorithmic responsibility effort collaborate not only with our other product and research teams, but also with our editorial teams and other domain experts, to make sure we understand the medium-specific challenges inherent to music, podcasting, and other domains. Cross-functional collaboration with our legal and privacy teams ensures that impact assessments are consistent with our strict privacy standards and applicable laws.
External collaboration
A crucial piece of the puzzle is learning from other researchers and practitioners. We contribute to the wider community by publishing our algorithmic responsibility research, sharing lessons and best practices, and facilitating collaborations with external partners. Making our work public is an important way of fostering informed conversation and pushing the industry forward.
How we expanded our efforts
Leveraging the approach above, we have (and continue to) evolve and expand our work to create a platform that’s safer, more fair, and more inclusive for listeners and creators:
We built out the capacity of our team to centrally develop methods and track our impact. This core team of qualitative and quantitative researchers, data scientists, and natural language processing specialists assists product and policy teams across the company to assess and address unintended harmful outcomes, including preventing the exacerbation of existing inequities through recommendations.
We helped create an ecosystem across the company for advancing responsible recommendations and improving algorithmic systems. This includes launching targeted work with specific product teams, as well as collaborations with machine learning, search, and product teams that help to build user-facing systems.
We introduced governance, centralized guidance and best practices to facilitate safer approaches to personalization, data usage, and content recommendation and discovery. This also creates space to further investigate inequitable outcomes for creators and communities by providing guidelines and best practices to mitigate algorithmic harms.
How we use algorithmic impact assessments at Spotify
Academic and industry researchers recommend auditing algorithmic systems for potential harms to enable safer experiences. Based on that groundwork, we’ve established a process for algorithmic impact assessments (AIA) for both music and podcasts. These internal audits are an essential learning tool for our teams at Spotify. But AIAs are just one part of putting algorithmic responsibility into practice. AIAs are like maps, giving us an overview of a system and highlighting hotspots where product adjustments or research into directional changes are needed.
Owning the product and the process
Our AIAs prompt product owners to review their systems and create product roadmaps in order to consider potential issues that may impact listeners and creators. The assessments are not meant to serve as formal code audits for each system. Instead, the assessments call attention to where deeper investigation and work may be needed, such as product-specific guidance, deeper technical assessment, or external review. Just like products, impact assessments, auditing methods and standards also need iteration following a first phase rollout. Through further refinement and piloting, we expect to continue to learn how the impact assessment process and translation between cross-functional teams can be improved.
Increasing accountability and visibility
For product owners, performing an assessment not only reinforces best practices leading to actionable next steps, it also increases accountability since audits are shared with stakeholders across the organization and work is captured and prioritized on roadmaps. Taken together, AIAs help us see where we have the most work to do as a company, including added visibility into teams’ product plans. Along with helping teams outline and prioritize their work, assessments also help establish roles and responsibilities. The AIA process includes the appointment of formal product partners to work with the core algorithmic responsibility team.
Results so far
In less than a year, we’ve educated a significant number of Spotifiers and assessed more than a hundred systems across the company that play a role in our personalization and recommendation work. The Spotify experience is produced by the interactions of many systems, from models that recommend your favorite genre of music to specific playlists. As a result of these efforts, we’ve:
Established formal work streams that led to improvements in recommendations and search
Improved data availability to better track platform impact
Reduced data usage to what is actually necessary for better product outcomes for listeners and/or creators
Helped teams implement additional safety mechanisms to avoid unintended amplification
Informed efforts to address dangerous, deceptive, and sensitive content, as described in our Platform Rules
Now that we have added AIAs as another tool for our teams to use, what has the process taught us about operationalizing algorithmic responsibility in practice?
Five lessons from implementing algorithmic impact assessments
AIAs will continue to help us understand where there is new work to be done and allow us to improve our methods and tools. And while direct answers to product questions are not always available — neither in the research literature nor in commercially available tooling — we would like to share five lessons that emerged from the process of implementing assessments.
1. Turning principles into practice requires continual investment
Operationalizing principles and concepts into day-to-day product development and engineering practicesrequires practical structures, clear guidance, and playbooks for specific challenges encountered in practice. This work will never be “done.” As we continue to build new products, update our existing products, and make advancements in machine learning and algorithmic systems, our need to evaluate our systems at scale will continue to grow. In some cases, we will need new tools and methods beyond what is currently available in either the research or industry communities around responsible machine learning. For others, we will need to find new ways to automate the work we do to reduce the burden on product liaisons.
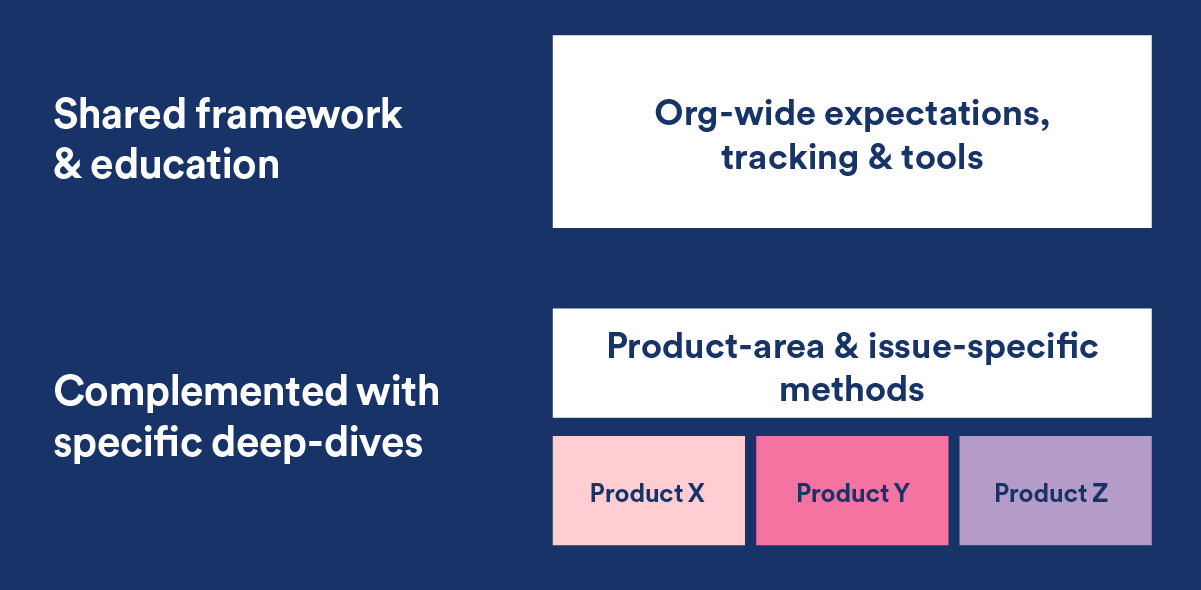
2. Algorithmic responsibility cannot just be the job of one team
It is critical that algorithmic responsibility efforts are not owned by just one team — improvement requires both technical and organizational change. Responsibility efforts require that every team understand their roles and priorities. We’ve focused on organizing people and processes as well as providing organizational support, including creating space for more than machine learning. This effort has enabled us to increase transparency and help make algorithmic responsibility ‘business as usual’ for hundreds of Spotifiers across the company.
3. There is no singular ‘Spotify algorithm’, so we need a holistic view
Product experiences depend on the interdependence of many systems, algorithms, and behaviors working together. This means that assessing and changing one system may not have the intended impact. Coordination across teams, business functions, and levels of the organization is essential to create a more holistic view of where there might be critical dependencies and methods to track across different types of systems.
4. Evaluation requires internal and external perspectives
Internal auditing processes like AIAs are complemented by external auditing, including work with academic, industry, and civil society groups. Our team collaborates with and learns from expertsin domains beyond just design, engineering, and product. Our work with Spotify’s Diversity, Inclusion & Belonging and editorial teams are crucial, as is our partnership with members of the Spotify Safety Advisory Council. We also work with researchers from disciplines such as social work, human-computer interaction, information science, computer science, privacy, law, and policy. Translation between disciplines can be challenging and takes time, but is crucial and worth the investment.
5. Problem solving requires translation across sectors and organizational structures
Timelines between product development and insights from the field can be very different, which means that it is crucial to set expectations that not all algorithmic impact problems can be easily ‘solved,’ especially not by technical means. Assessment efforts should be informed by research on what matters in a specific domain (e.g. representation in music or podcasting, as well as the influence of audio culture on society), but also internal research on how to best create structure and support within your existing organizations and development processes. That is why we are actively investing in data, research, and infrastructure to be able to track our impact better, and sharing best practices with others across academia and industry. Our team also translates insights and learning through the broader field, including participation in academic and industry conferences and workshops, as well as through formal collaboration with independent researchers.
Where we are going
This is an ever-evolving field, and as members of this industry, it is our responsibility to work collaboratively to continue to identify and address these topics. Just as content itself and the industry that surrounds it continues to evolve, our work in algorithmic impact will be iterative, informed, and focused on enabling the best possible outcomes for listeners and creators.
To learn more about our research and product work, visit: research.atspotify.com/algorithmic-responsibility.
We are grateful to members of the Spotify Safety Advisory Council for their thoughtful input and ongoing feedback related to our Algorithmic Impact Assessment efforts.