Are You a Dalia? How We Created Data Science Personas for Spotify’s Analytics Platform

On Spotify’s Analytics Platform, we’re dedicated to building products that empower data practitioners to discover, analyze, and share insights — think notebooks, dashboards, and metrics. To create solutions that connect with our users, it’s critical for us to first understand who our users are. However, the evolving landscape of data roles presents a challenge.
In the past decade, we’ve observed an industry trend toward consolidating diverse roles under the Data Scientist umbrella. Yet not all data scientists have the same needs, and many employees working with data don’t even hold that title. While we adopted the term “data practitioner” as a workaround, it proved too broad to effectively guide our product development. So we built a framework to better understand who our data practitioners are. Enter personas.
To create personas, we took a mixed-methods approach, meaning we blended qualitative insights from in-depth interviews with quantitative analysis of employee data, allowing us to identify clusters of shared behaviors. We pushed for personas that were both interpretable and applicable so that product leaders could easily grasp them and use them to make tactical decisions.
This work resulted in six distinct personas that compose the data practitioner universe at Spotify, along with several learnings relevant to all insights practitioners.
Curious to see the personas? Skip to the end!
What did we learn along the way?
- Don’t just facilitate collaboration between Data Science and Research by lowering barriers. Instead, erase the barriers between data science and user research.
While mixed-methods research sometimes involves one method following another, we found success in having an iterative loop — using the qualitative work to inform the quantitative work, then the quantitative work to inform the qualitative work, and so on, until we’re satisfied with the end result. After a series of user interviews, we created our first version of the quantitative rules that would inform persona classification. We then continued to interview additional users to help us validate our classifications, add depth to our knowledge of the archetypes, and break ties to improve the rules we were developing. This became an iterative process of qualitative and quantitative validation, where each user interview we conducted resulted in either validation or tweaks to the proposed quantitative rules. - Don’t just share findings and send the deck around. Create momentum by openly discussing tailored recommendations with your stakeholders and connecting them to product initiatives.
A common challenge to foundational work can be activating the work and actioning on the findings. Stakeholders may be wondering: What do we do now that we know this?
To encourage action, we created dedicated slides in our share-outs highlighting the personas for each team to focus on. These recommendations were open for discussion but gave a good starting point to engage with the content. We also offered suggestions on how the personas could inform future product strategy.
With these slides as primers, we witnessed our teams learn a common language and understanding of who we were building for. Teammates used the new vocabulary to talk about our users during sprints and planning. Over the first quarter, the framework went from being a conversation starter to becoming the foundation for how we set goals and metrics. - Don’t just write the research plan and go off to work. Engage with your stakeholders from start to finish.
For anything but the smallest questions, data scientists and user researchers have a tendency to withdraw into the research process and emerge at last with final answers, detached from changing requirements during the research period. Keeping our stakeholders involved throughout the project reduced this risk.
Before kicking off the project, we aligned on the background and scope of the work and remained open to stakeholder feedback. To easily follow along during the project, we created a dedicated Slack channel for the research, to share updates and anecdotes on the fly. We also opened user interviews for observation across the entire product area, regardless of squad and role.
We also found that foundational work with longer timelines benefitted from sharing early learnings at key milestones. Midway through the project, we shared “Postcards from the Field” — raw, unsynthesized qualitative data that kept stakeholders up to speed with the research. We also shared previews of the personas with our product area leads at later milestones to gather feedback. - Don’t take a complex quantitative approach for its own sake. Keep your approach simple and clear.
We used a rule-based approach to classify Spotifiers into personas. (For example: “Employee has used dashboarding tools more than 90% of data scientists.”) Keeping the approach simple made our results easily interpretable, transparent, and trustworthy to our stakeholders. - Don’t just move on to another project. Instead, find opportunities to extend the work beyond the original scope of the project.
To ensure the personas would be used among our teams going forward, we built a dataset to classify every internal user at Spotify into a persona on a daily basis. This dataset has not only become helpful for recruitment for future initiatives, but also adds more color to our product area metrics. We are now able to segment all our key results by these persona types and explore user behaviors for each group.
Because this work is large in scale and built foundational knowledge across our product area, we wanted to make sure that the outcome was easy to refer to and share. To make the personas more memorable, we collaborated with our design partners to create Spotify-approved illustrations, adding a face to each one. Building strong visual artifacts encouraged greater longevity and shareability.
Your turn: Which persona are you?
If you work in the field, you may be asking yourself: So which data persona am I?
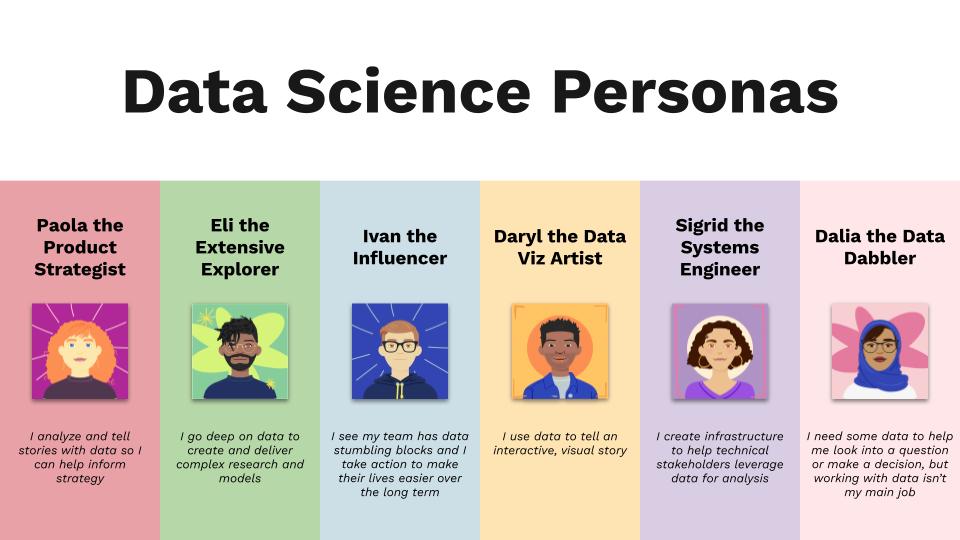
Paola the Product Strategist: Paolas are the most common data science persona at Spotify. They analyze and tell stories with data to help inform strategy. They typically partner closely with non-technical stakeholders. Outside Spotify they hold titles like Product Analyst or Data Scientist, Analytics.
Eli the Extensive Explorer: Elis are the second-most-common persona type at Spotify. These are data scientists, machine learning engineers, or research scientists who go deep on data to deliver complex research and models. This persona brings together the traditional data science role with MLE and similar roles.
Ivan the Influencer: Ivans are leaders who support their data teams and have influence over best practices and processes. They may be team leads or managers.
Daryl the Data Viz Artist: Daryls use data to tell interactive, visual stories and spend most of their time creating dashboards to share with non-technical stakeholders. This role is a niche at many companies and may not even have its own title.
Sigrid the Systems Engineer: Sigrids are engineers who create infrastructure to help technical stakeholders leverage data for analysis. Data Engineer or Analytics Engineer are common titles.
Dalia the Data Dabbler: Dalias are not data scientists by title but work with data to perform analysis or make decisions. They typically explore straightforward data questions, often with technical support from the other personas.
–
Did one of the personas resonate with you? We found that most Spotify data practitioners related closely to one persona, but some employees reported fitting a hybrid of two personas.
Tags: Data, engineering culture